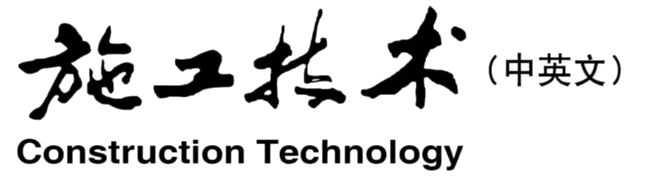

DOI: 10.7672 / sgjs2025070129
Aiming at the problem of serious cutter wear when the shield machine passes through the rockstratum, based on the excavation examples of sand⁃rock composite stratum and medium⁃hard rock stratumin the shield tunnel project of the right line of Nanjing Heyan Road Cross⁃River Channel, the wear of theinserted⁃tooth hob is measured, its wear law is analyzed, and the BP neural network is used to fit andpredict the measured cutter tooth collapse. The results indicate that the most influential factors on thewear of the inserted⁃tooth hob in the sand⁃rock composite stratum are penetration and propulsion speed,while in the medium⁃hard rock stratum, it is the cutterhead torque. In the process of shield machinetunneling from sand⁃rock composite stratum to medium⁃hard rock stratum, the number of cutter teeth ofthe inserted⁃tooth roller cutter gradually increases, and the proportion of cutter teeth defect increases from28% to 86%. At the same time, the eccentric wear of the roller cutter is becoming increasingly serious.After BP neural network training, a neural network prediction model with a smaller mean square error canbe obtained. The amount of model training data is directly related to the accuracy of the BP neuralnetwork model in predicting cutter tooth collapse. In the case of smaller training data (56 groups), the prediction error rate of cutter tooth collapse in sand⁃rock composite strata is 45%. The average predictiongap of cutter tooth collapse in medium⁃hard rock strata with more training data (135 groups) is 8.03%.It is recommended to control a smaller cutterhead speed to reduce wear when tunneling in hard rockformations. Meanwhile, more sample training data should be measured when the tool wear is actuallypredicted, thereby improving the prediction accuracy.